일 | 월 | 화 | 수 | 목 | 금 | 토 |
---|---|---|---|---|---|---|
1 | 2 | 3 | 4 | 5 | ||
6 | 7 | 8 | 9 | 10 | 11 | 12 |
13 | 14 | 15 | 16 | 17 | 18 | 19 |
20 | 21 | 22 | 23 | 24 | 25 | 26 |
27 | 28 | 29 | 30 |
Tags
- 웨크롤링
- OpenAI
- 티어구조
- 블루그린배포
- Python
- 쳇지피티
- 3티어아키텍처
- web crowling
- 웹크롤링
- java17
- MSA
- 카나리배포
- AI
- API
- chatGPT
- 클라우드네이티브
- temurin
- chaosengineering
- 티스토리챌린지
- 레이어패턴
- DALL-E
- 오블완
- xmlschema
- 책임분리
- 무상태
- 12factorapp
- JSON
- 포트바인딩
- ci_cd
- WSL
Archives
- Today
- Total
Nacho
Pandas 데이터프레임 Concat(), Merge() 본문
반응형
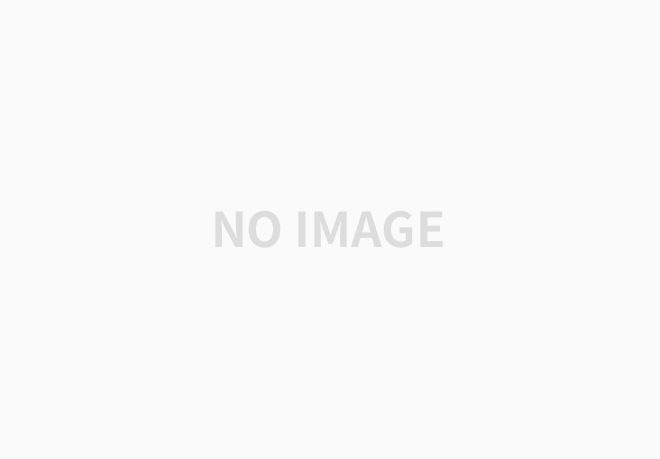
자. 데이터프레임을 주물러보자.
pd.concat()
- axis 값을 통해 결합 방향을 설정한다.
- join을 사용하여 결합 방식을 설정한다.
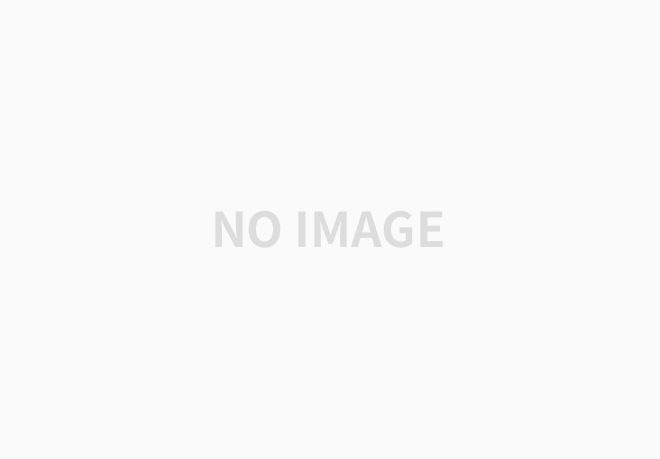
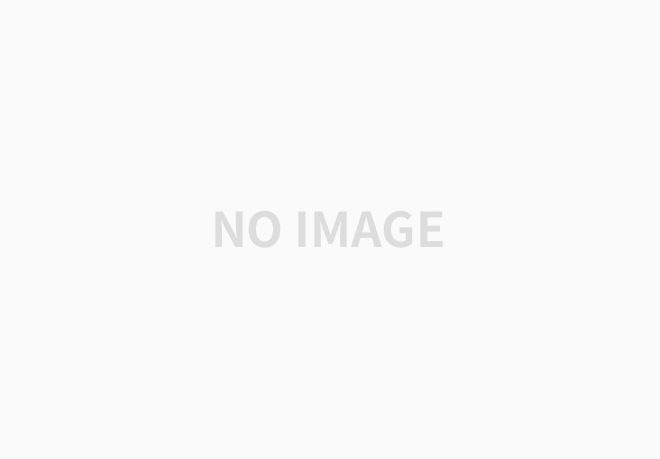
🔍 두 DataFrame을 사용하여 결합 방식을 살펴보자.
- axis = 0, 행으로 결합 (세로 방향), 우선 세로 방향으로 데이터가 추가하자.
- 공통되는 열을 기준으로 표를 재배치해본다면 다음과 같다.
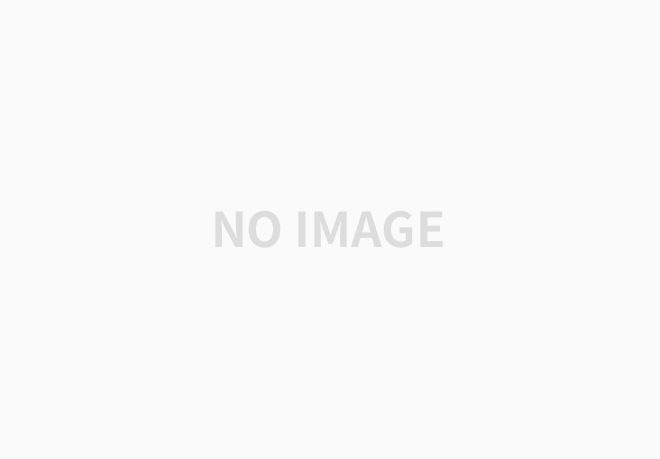
표가 생긴게 이상하지만 중간 과정이라고 생각하면 이해가 쉬워진다
- join = 'inner' 공통된 열만 합치기.
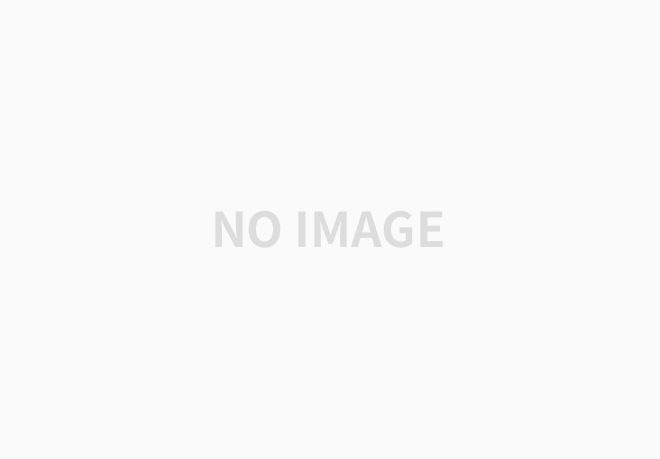
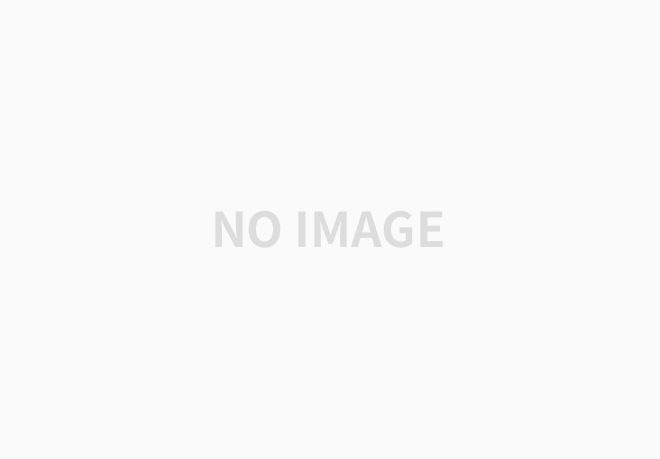
- join = 'outer' 모든 열 합치기. 남은 빈칸을 결측치로 채워 반환한다.
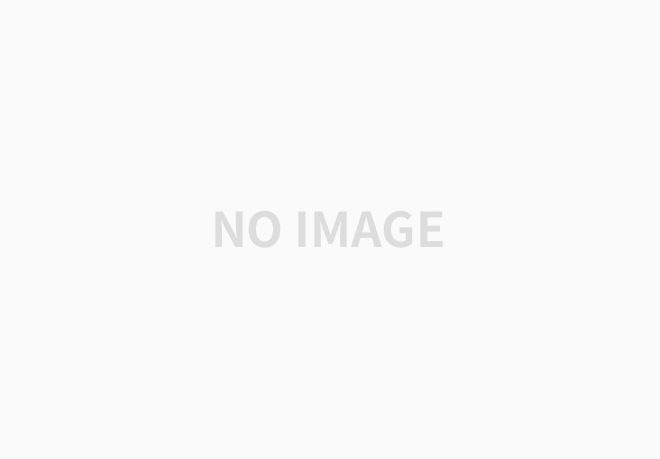
- axis = 1, 열로 결합 (가로 방향), 우선 가로 방향으로 데이터를 추가하자.
- 공통되는 행을 기준으로 표를 재배치해본다면 다음과 같다.
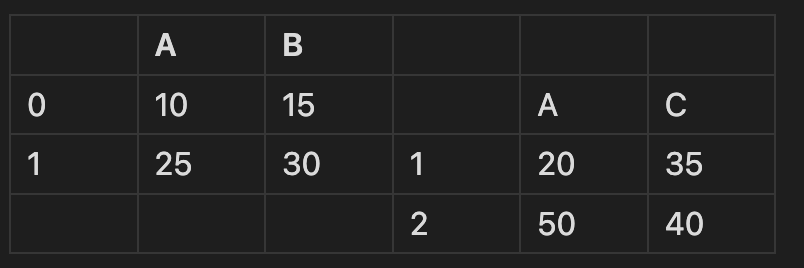
표가 생긴게 이상하다. 맞다.
- join = 'inner' 공통된 행만 합치기.
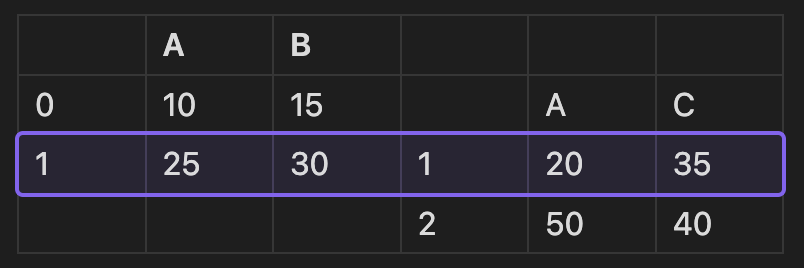
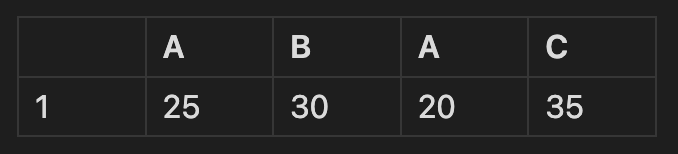
- join = 'outer' 모든 행 합치기. 남은 빈칸을 결측치로 채워 반환한다.
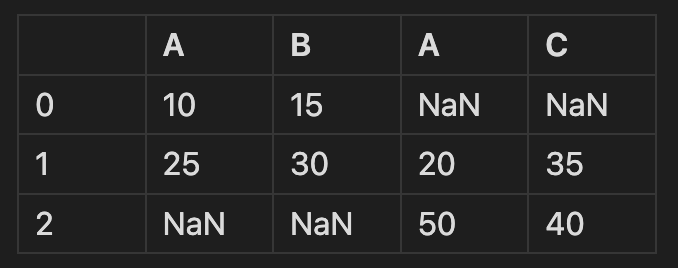
pd.merge()
- 기준이 되는 column로 병합.
Example로 이해하는 것이 쉽다.
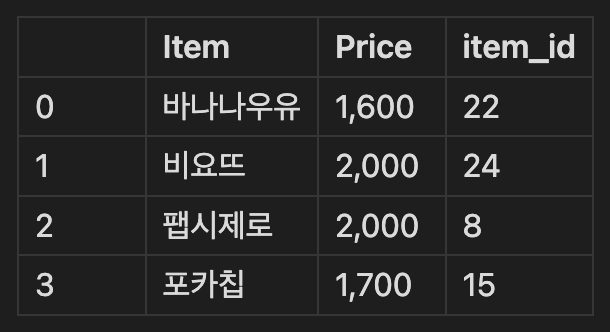
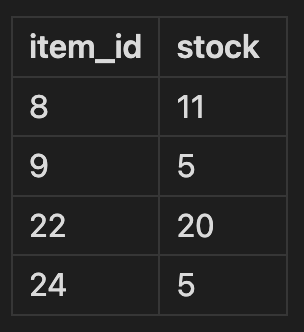
🔍 두 DataFrame을 사용하여 병합 방식을 살펴보자.
첫 번째 데이터는 물건들의 이름, 가격, 물품 번호가 들어있고, 두 번째 데이터는 물품 번호, 재고 상태를 나타낸다.
이 두개의 표를 하나의 표로 만들기 위해서 item_id column을 기준으로 합쳐야 한다는 직관이 들 것이다. 그것이 merge()의 역할이다.
- how = 'inner', on='item_id'
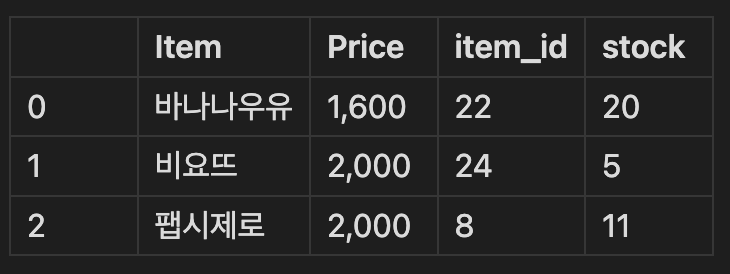
- how = 'outer', on='item_id'
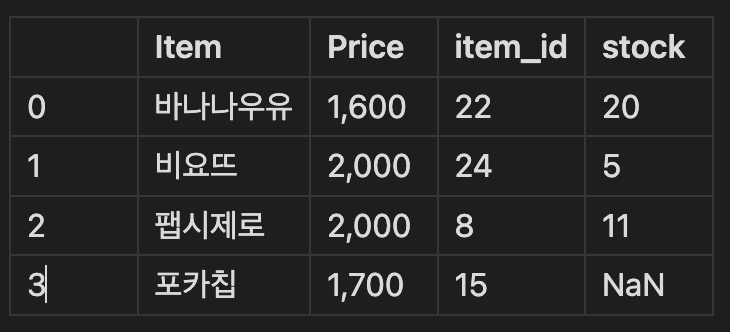
복습 문제
sales = pd.read_csv("https://raw.githubusercontent.com/DA4BAM/dataset/master/ts_sales_simple.csv")
products = pd.read_csv("https://raw.githubusercontent.com/DA4BAM/dataset/master/ts_product_master.csv")
stores = pd.read_csv("https://raw.githubusercontent.com/DA4BAM/dataset/master/ts_store_master.csv")
sales에 금액(Amt) 변수를 추가하시오.
- Amt = Qty * Price
sales_p = pd.merge(sales,products,how='inner',on='Product_ID')
sales_p['Amt'] = sales_p['Qty'] * sales_p['Price']
sales_pc = pd.merge(sales_p,stores,how='inner',on='Store_ID')
sales_pc.head()

2) City별 매출액
sales_pc.groupby('City')[['Amt']].sum().T

3) City별 카테고리별 매출액
sales_pc.groupby(['City','Category'],as_index=False)[['Amt']].sum()
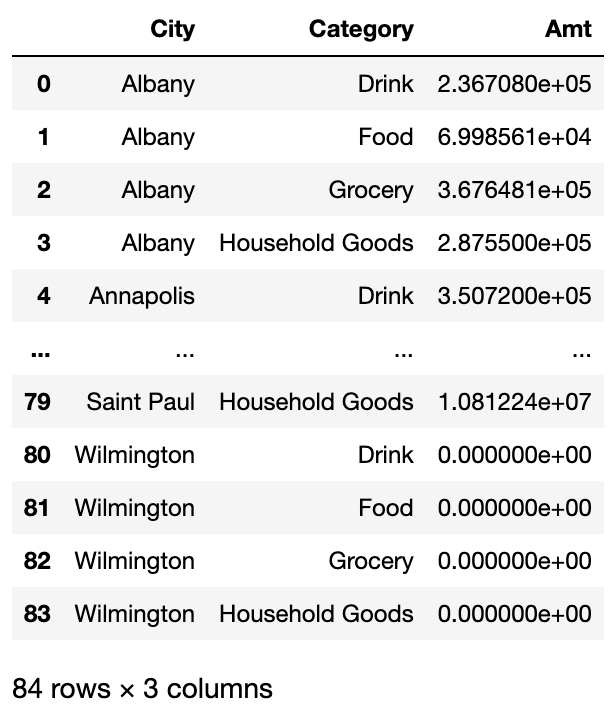
4) 매출액이 가장 높은 주(state) top 3
sales_pc.groupby('City',as_index=False)[['Amt']].sum().sort_values(by='Amt',ascending=False)[:3]
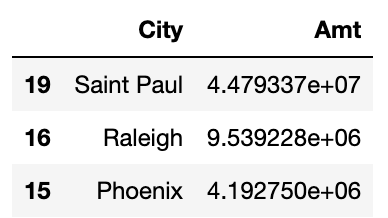
- 2013년 1월 세째 주 각 도시별 subCategory별 금액 비교
- 2013년 1월 세째 주
- sales['Date']를 날짜 타입으로 변환
- week 추가
- sales에서 week가 3인 데이터 뽑기
- 각 도시별 subCategory별
- 1의 결과에서...
- stores와 inner merge
- products와 inner merge
- 도시별 subCategory별 금액
- Qty * Price로 Amt 추가
- groupby
- 좀더 보기 좋게! pivot + heatmap
- pivot
- heatmap
- 2013년 1월 세째 주
sales['Date'] = pd.to_datetime(sales['Date'])
sales['Week'] = sales['Date'].dt.isocalendar().week
sales_3_week = sales.loc[sales.Week == 3]
sales_3_week.head()
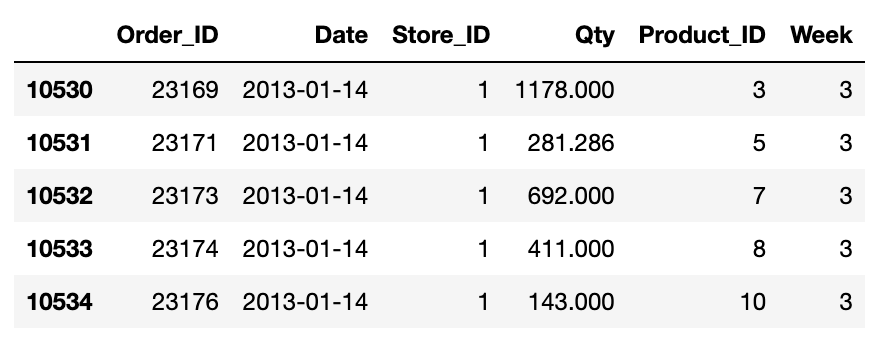
sales_3_s = pd.merge(sales_3_week,stores,how='inner')
sales_3_sp = pd.merge(sales_3_s,products,how='inner')
sales_3_sp['Amt'] = sales_3_sp['Qty']*sales_3_sp['Price']
sales_3_sp.head()
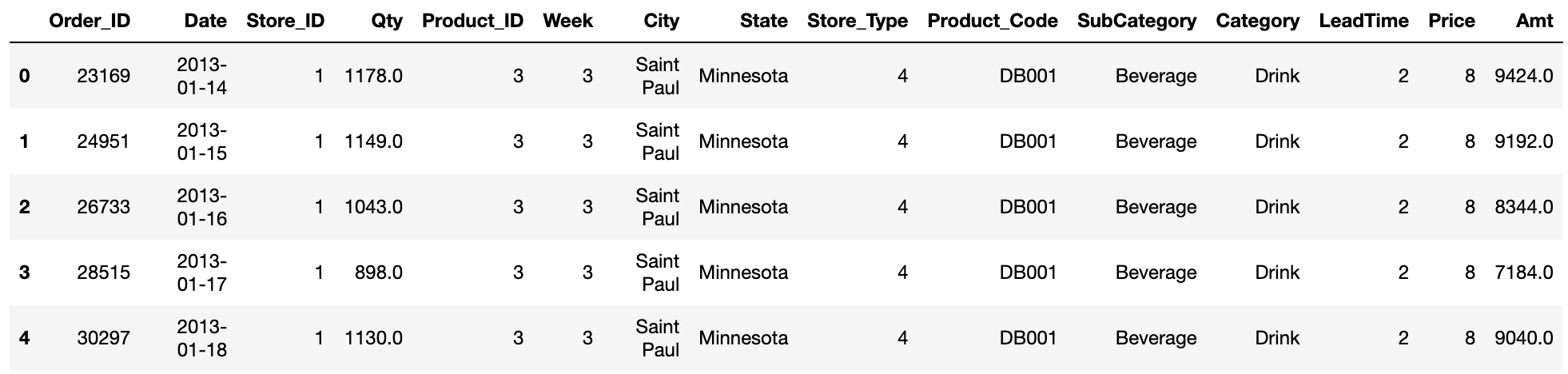
Amt_for_3week_SubCat = sales_3_sp.groupby(['City','SubCategory'],as_index=False)['Amt'].sum()
Amt_for_3week_SubCat.head()
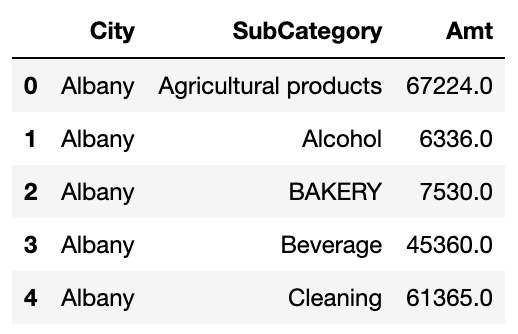
Amt_for_3week_SubCat = Amt_for_3week_SubCat.pivot('City','SubCategory','Amt')
Amt_for_3week_SubCat
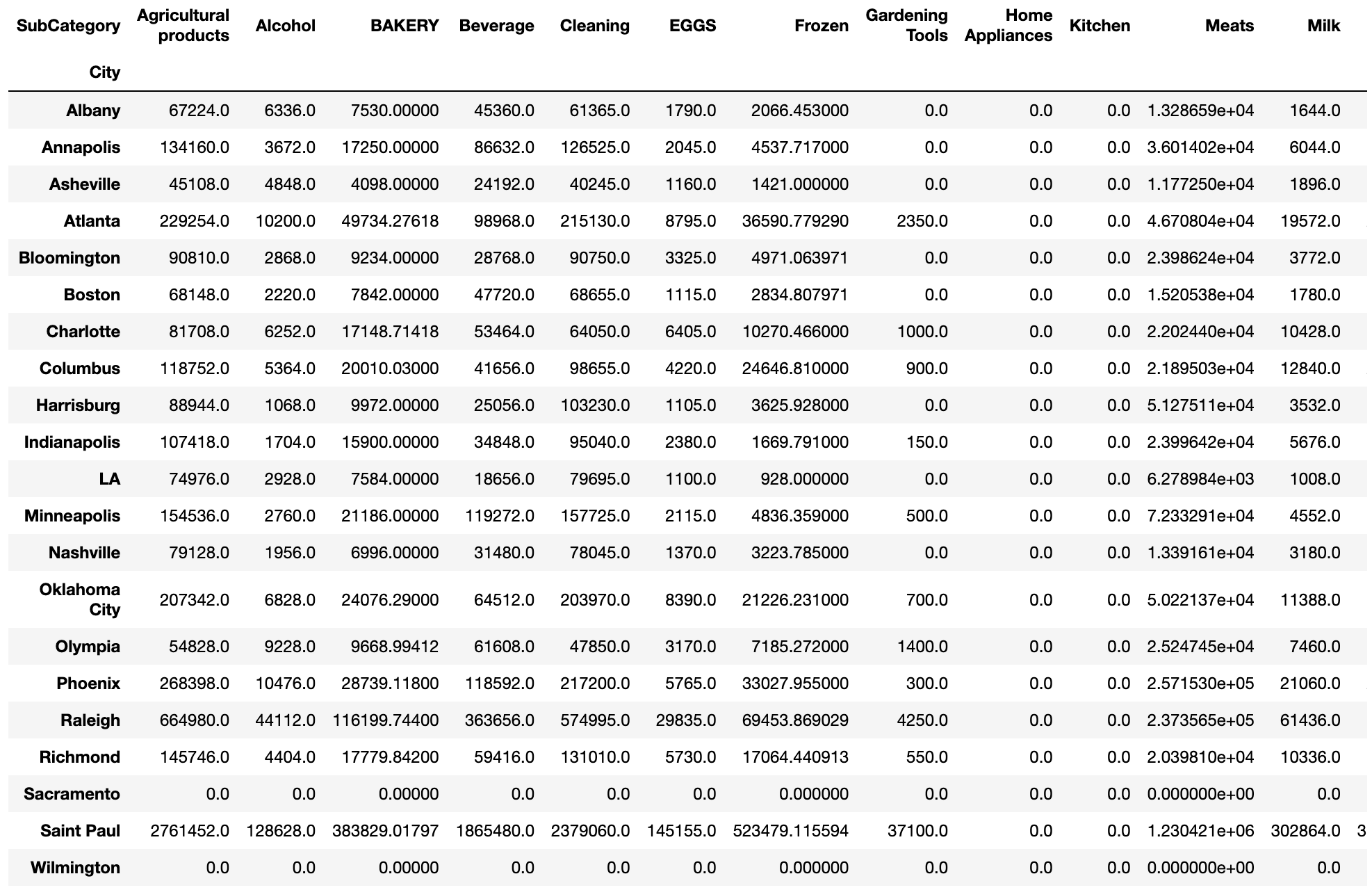
sns.heatmap(Amt_for_3week_SubCat)
plt.show()
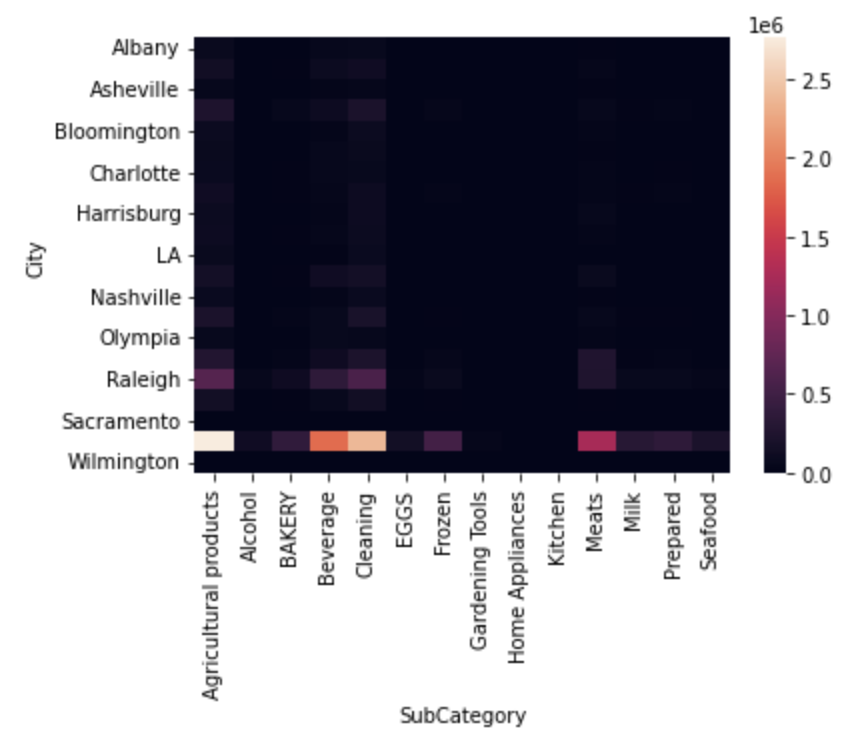
반응형
'Python' 카테고리의 다른 글
(Python) 모평균 검정 (t-test, ANOVA) (0) | 2024.02.28 |
---|---|
(Python) 평균 추청과 신뢰구간 (모평균 추청, 중심극한정리) (0) | 2024.02.28 |
Chapter 08. Python Pandas 기초(2) (0) | 2024.02.23 |
Chapter 07. Python Pandas 기초(1) (0) | 2024.02.23 |
Chapter 06. Python Numpy 기초 (0) | 2024.02.23 |